Synthetic Data in AI Training: Overcoming Key Challenges
In the world of artificial intelligence (AI), data is often considered the lifeblood of model training. However, obtaining high-quality, real-world data presents numerous challenges. From privacy concerns to data scarcity and high costs, AI developers face significant obstacles in sourcing the data they need. Enter synthetic data — a game-changing solution that has emerged as a powerful tool for overcoming these challenges. In this article, we will explore the growing role of synthetic data in AI training, its applications, benefits, challenges, and future potential.
I. What is Synthetic Data?
Defining Synthetic Data for AI Models
Synthetic data refers to artificially generated data designed to replicate the patterns and characteristics of real-world data. This data is used in AI model development to simulate various conditions that may be difficult or impossible to capture with real-world data. Synthetic datasets offer AI developers a scalable, controlled, and versatile approach to creating training datasets without the constraints of sourcing real-world data.
How is Synthetic Data Created?
There are several methods for generating synthetic data used in AI training, each with its own strengths:
Data Augmentation for AI Models
Data augmentation involves applying transformations (e.g., rotation, flipping, scaling) to existing datasets. For instance, rotating images in a dataset of cars can generate new data points to train image classification models.
Simulation-Based Data Generation
In industries like autonomous driving and robotics, synthetic data is often generated through simulated environments. These simulations recreate real-world scenarios, such as different weather conditions or traffic patterns, to help AI models train on diverse situations.
Generative Models for Data Creation (e.g., GANs)
Generative Adversarial Networks (GANs) are a popular method for creating synthetic data. GANs consist of two neural networks: a generator that creates synthetic data and a discriminator that evaluates it. This adversarial process helps improve the realism of generated data, especially in applications like image creation or natural language processing.
II. Why Synthetic Data is Key to AI Model Development
Addressing Data Scarcity in AI
One of the biggest challenges AI faces is the scarcity of high-quality data. Many industries, such as healthcare, finance, and retail, face difficulties sourcing enough real-world data to train their models effectively. Synthetic data provides a rich, abundant source of training data, addressing the data scarcity problem.
The Cost Advantage of Synthetic Data
Data collection and annotation can be expensive and time-consuming. In fields like autonomous driving or medical research, gathering data requires specialized equipment and human resources. Synthetic data can be produced at a fraction of the cost, enabling businesses to scale AI model training without incurring high expenses.
III. Real-World Applications of Synthetic Data
Autonomous Vehicles and Synthetic Data
The autonomous vehicle industry has been a pioneer in using synthetic data. Self-driving cars need vast amounts of data to train their AI systems on different road conditions, weather patterns, and traffic scenarios. While collecting such data in the real world is costly and time-consuming, synthetic data provides a practical solution. By using simulated driving environments, companies can generate diverse training datasets without the logistical constraints of real-world data collection.
Synthetic Data in Healthcare: Preserving Privacy
In healthcare, privacy laws such as HIPAA (Health Insurance Portability and Accountability Act) in the U.S. restrict access to real patient data, making it difficult to use for AI training. Synthetic medical data addresses this challenge by offering anonymized datasets that help train AI models for tasks like disease diagnosis, drug discovery, and personalized medicine. For instance, synthetic datasets have been used to train models for detecting cancer in medical imaging without exposing any real patient data.
Facial Recognition AI Models and Security
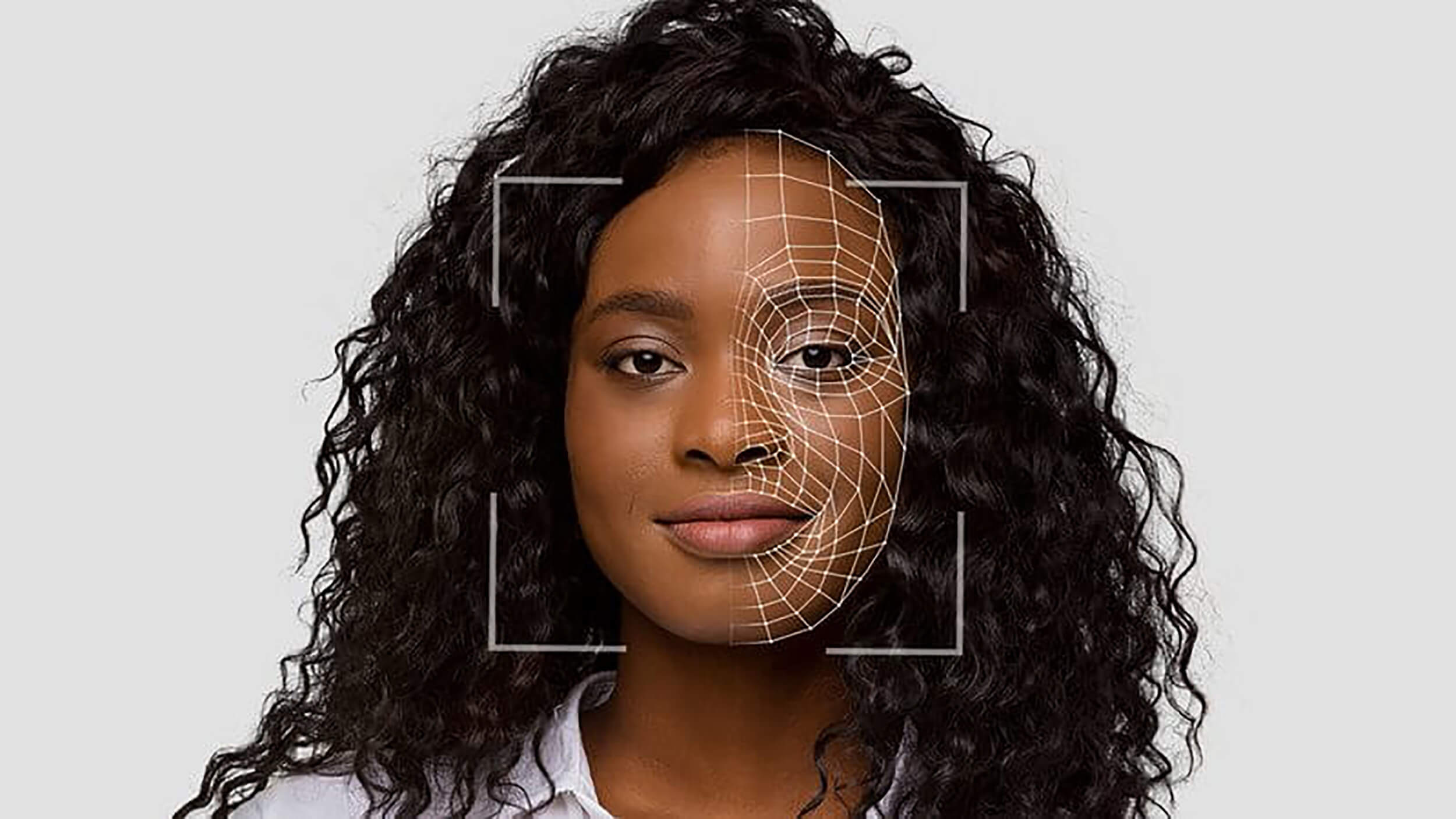
Synthetic data is also proving invaluable for training facial recognition systems. To create diverse and representative datasets for these systems, companies need a wide variety of images, including different lighting conditions, facial expressions, and demographics. With synthetic data, businesses can generate millions of varied facial images to train facial recognition algorithms without relying on personal or sensitive real-world data.
IV. Benefits of Synthetic Data in AI Training
Enhancing AI Performance with Diverse Data
Synthetic data improves AI model performance by enhancing their ability to generalize across a wide range of scenarios. For example, a self-driving car trained with synthetic data is better equipped to handle rare and extreme driving conditions, ensuring safer performance on the road.
Cost and Time Efficiency in Model Development
Synthetic data reduces the time and cost associated with gathering real-world data. The ability to generate large volumes of data quickly helps businesses speed up the development of AI models and bring AI-driven products and services to market faster.
Scalability of AI Training Datasets
Synthetic data allows for the rapid scaling of AI training datasets. As AI applications become more complex, the ability to generate vast datasets without relying on real-world data is crucial for keeping up with demand.
V. Challenges of Using Synthetic Data
Ensuring Data Realism for Accurate AI Models
The effectiveness of synthetic data depends on how well it mimics real-world conditions. If the synthetic data does not adequately represent the real world, it may lead to biased or inaccurate models. Ensuring high-quality synthetic data is one of the key challenges for AI developers.
Validating Synthetic Data for AI Use
Validating synthetic data for accuracy and relevance remains challenging. Since synthetic data isn’t always grounded in real-world observations, ensuring that it contributes to effective AI model training is crucial.
VI. The Future of Synthetic Data in AI Model Training
Advancements in Generative Models for Data
As generative models like GANs and VAEs (Variational Autoencoders) continue to improve, synthetic data will become even more realistic and applicable to a broader range of AI domains.
Combining Synthetic and Real-World Data for AI Training
The future of synthetic data lies in its integration with real-world data. By combining the strengths of both, AI models will become even more effective and efficient.
Conclusion
Synthetic data is quickly becoming a cornerstone of AI model training, offering solutions to the challenges of data scarcity, privacy concerns, and high costs. While it does have limitations, synthetic data holds enormous potential to revolutionize AI development, making it a crucial tool for businesses and researchers alike. By embracing synthetic data, organizations can accelerate AI innovation while ensuring their models are both scalable and diverse. As this field evolves, the possibilities that synthetic data unlocks for AI applications across industries are exciting and promising
Click here to know about the TOP 10 AI TOOLS FOR BEGINNERS !