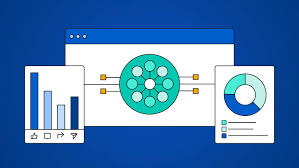
From Data to Ideas: AI content prediction
Introduction
Ever spent hours poring over analytics reports, only to feel stuck when deciding what to publish next? You’re not alone. With endless audience behavior data — from pageviews and bounce rates to social shares and comments — it’s easy to feel overwhelmed by numbers yet starved of actionable insights. Enter AI content prediction, the game‑changer that sifts through raw metrics and highlights the topics, headlines, and formats poised to drive real engagement. In this article, we’ll dive into how predictive content analytics works, explore the top tools to get started, and share best practices to supercharge your content strategy.
Why Data Alone Isn’t Enough
The Data Deluge
Imagine staring at a dashboard filled with dozens of charts and graphs. You see impressions, click‑through rates, time on page, and more—but which metric truly signals success? When metrics live in silos, it’s easy to chase vanity numbers instead of meaningful growth. Marketers often end up spinning their wheels, unsure which data points to prioritize for maximum impact
Human Limits in Pattern Recognition
Our brains are marvels of complexity, but they struggle to detect subtle patterns across thousands of data points. Cognitive biases can lead us to overvalue recent wins or ignore underperforming experiments, skewing our decisions. That’s where machine learning for marketing swoops in: by objectively analyzing vast datasets, AI reveals hidden correlations that human analysts might miss, empowering you to make data‑driven choices with confidence.
How AI Transforms Data into Predictive Insights
Fundamentals of Predictive Analytics
At a high level, predictive analytics uses statistical models and algorithms to forecast outcomes based on historical data. For content, this means feeding past performance metrics into algorithms that can estimate the likelihood of success for future topics. Techniques like regression analysis predict numerical outcomes (e.g., expected pageviews), while classification models tag content ideas as “high potential” or “low potential.”
Machine Learning Models for Content
- Supervised Learning: Algorithms learn from labeled examples—say, posts tagged as “viral” or “flop”—to map features like headline length, keyword usage, or publish time to engagement outcomes. Popular choices include Random Forests and Gradient Boosting Machines.
- Unsupervised Learning: Techniques like clustering group similar content ideas based on engagement patterns, revealing niche topics or underserved audience segments.
Key Data Inputs & Feature Engineering
Great predictions hinge on quality features. Common inputs include:
- Engagement Metrics: Click‑through rate (CTR), average session duration, bounce rate.
- Audience Behavior Data: Scroll depth, repeat visits, demographic signals.
- Social Signals: Shares, comments, sentiment analysis.
- External Factors: Seasonality, trending topics, competitor performance.
From Predictions to Actionable Ideas
Once your model surfaces high‑potential topics, translate them into creative angles. For example, if “AI in healthcare” shows a 75% predicted engagement lift, brainstorm a listicle like “5 Ways AI is Revolutionizing Patient Care.” Use clustering outputs to build content series, and let recommendation algorithms guide your editorial calendar.
Tools & Platforms to Get Started
- Platform A: A no‑code solution with drag‑and‑drop feature builders, automated model training, and built‑in A/B testing. Ideal for teams without data science resources.
- Platform B: Developer‑friendly APIs that integrate seamlessly with CMS platforms like WordPress or Contentful. Offers real‑time predictions and custom model deployment.
- Open‑Source Libraries: For hands‑on experimentation, libraries such as scikit‑learn, TensorFlow, and PyTorch let you prototype custom models. Combine with automation tools like Airflow or Prefect for reliable data pipelines.
Curious which tool fits your workflow? Explore our full comparison of the best AI content platforms to find your match.
Best Practices for AI‑Powered Content Strategy
- Start Small & Iterate
Pilot AI predictions on a single channel or topic cluster. Refine your approach before scaling across all content. - Maintain Clean, Centralized Data
Centralize metrics in a data warehouse or analytics platform to avoid duplicated, outdated datasets. - Foster Cross‑Functional Collaboration
Pair data analysts with content creators to ensure insights translate into engaging narratives. - Implement Continuous Feedback Loops
Feed actual performance back into your models for ongoing improvement and stronger content performance forecasting. - Prioritize Ethics & Transparency
Clarify how AI suggestions are generated to build trust with stakeholders and mitigate bias.
Measuring Success & Optimization
Track key metrics—engagement lift, time on site, conversion rate—and compare AI‑suggested content against human‑curated topics through A/B testing. Use insights to fine‑tune feature sets (e.g., adjust for seasonality) and tweak recommendation algorithms, ensuring your strategy evolves with audience preferences.
Future Trends in AI‑Driven Content
- Generative AI for Rapid Ideation: Tools like GPT‑style models can draft outlines, headlines, and even full drafts in seconds.
- Hyper‑Personalization at Scale: Real‑time content adaptations based on individual reader preferences.
- Advanced Semantic Understanding: Deep learning models that grasp nuance, context, and tone, enabling more authentic, human‑sounding content.
Conclusion & Next Steps
From overwhelming data to clear, actionable ideas, AI content prediction empowers you to create content that truly resonates. Ready to elevate your strategy and drive measurable results?
👉 Registration link: https://lu.ma/hkvfuw3f
If you’re new to predictive analytics, kick off your learning with our beginner’s guide here, and let AI steer your content roadmap toward success!
Stay connected with us on HERE AND NOW AI & on: